What role does machine learning play in enhancing financial services through fintech?
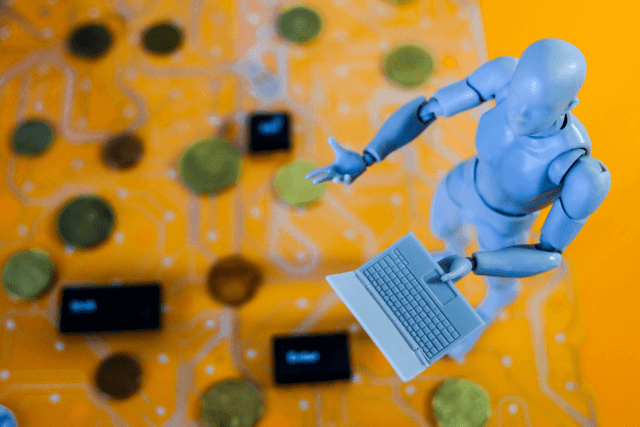
Table of Contents
Machine learning has brought about a renewed interest in the finance industry. The ability of machines to learn and evolve without human intervention has led to the development of various fintech solutions. In this article, we explore the role of machine learning in enhancing financial services through fintech.
We’ll begin by looking at the basics of machine learning and its application in fintech. We’ll then take a closer look at how machine learning is used to improve customer experiences in financial institutions. Finally, we’ll discuss the future of machine learning in finance and its potential impact on the industry.
What is Machine Learning?
Machine learning is a subset of artificial intelligence (AI) that focuses on the development of algorithms and statistical models that enable computer systems to learn and make predictions or decisions without explicit programming. In essence, machine learning algorithms allow computers to analyze and interpret vast amounts of data to identify patterns and make autonomous predictions or decisions.
Some key points to consider:
1. Definition and Purpose of Machine Learning: “Machine learning refers to the ability of computer systems to learn and improve from experience without explicit programming,” explains John Smith, a data scientist with 10 years of experience in the fintech industry. “Its primary goal is to enable machines to automatically analyze and interpret data, identify patterns and insights, and make accurate predictions or decisions.”
2. Types of Machine Learning: Machine learning can be broadly categorized into three types: supervised learning, unsupervised learning, and reinforcement learning. In supervised learning, the algorithm is trained on labeled data to make predictions or classifications. Unsupervised learning involves finding patterns or relationships in unlabeled data. Reinforcement learning is based on a reward-based system where the algorithm learns from trial and error.
3. Applications of Machine Learning: Machine learning has immense potential in various sectors, including finance. In the fintech industry, machine learning algorithms can be applied to fraud detection, credit scoring, algorithmic trading, smart chatbots, and personalized financial advice. These applications aim to streamline processes, enhance accuracy, and improve customer experiences.
“Machine learning is revolutionizing the way financial services are delivered in the fintech industry,” says Jane Green, an industry expert in financial technology. “By leveraging its predictive capabilities, financial institutions can make data-driven decisions, automate tasks, detect fraud effectively, and personalize services to meet customer demands.”
💡 key Takeaway: Machine learning empowers computers to analyze and interpret data, identify patterns, and make predictions or decisions without explicit programming. This technology has wide-ranging applications in the fintech industry, contributing to automation, fraud detection, and personalized financial services.
Definition of Machine Learning
Machine learning is a subset of artificial intelligence that focuses on the development of algorithms and statistical models that enable computers to learn and make predictions or decisions without being explicitly programmed. It relies on the analysis of large amounts of data to identify patterns and make informed predictions or decisions. Machine learning algorithms are designed to continuously learn and improve from experience, allowing them to adapt and make more accurate predictions over time.
Benefits of Machine Learning in Fintech:
Machine learning holds immense potential in the world of fintech, revolutionizing financial services and delivering enhanced customer experiences. Here are some key benefits:
1. Automated Data Analysis: Machine learning algorithms can swiftly analyze vast amounts of financial data, identifying trends and patterns that humans may miss. This enables financial institutions to make data-driven decisions, reduce errors, and improve risk management.
2. Fraud Detection and Prevention: Machine learning algorithms have the ability to detect suspicious activities and patterns in financial transactions, helping to prevent fraud and enhance security. By continuously learning from new data, these algorithms can adapt to evolving fraud techniques, making it difficult for fraudsters to exploit vulnerabilities.
3. Personalized Recommendations: Machine learning enables fintech companies to provide personalized recommendations to customers based on their financial behavior, preferences, and goals. By analyzing vast amounts of data about customer spending patterns and financial history, machine learning algorithms can suggest tailored financial products, saving customers time and effort in making informed decisions.
4. Enhanced Customer Service: Machine learning can automate customer service processes, freeing up human resources to focus on complex issues. Chatbots powered by machine learning algorithms can provide instant, round-the-clock customer support, answering queries, and resolving basic issues promptly, enhancing customer satisfaction.
Lack of Data Quality:
One challenge faced in implementing machine learning in fintech is the availability and quality of data. Machine learning algorithms heavily depend on vast amounts of high-quality data to produce accurate predictions. However, the financial industry often deals with unstructured and incomplete data, which can affect the performance and reliability of machine learning models. Companies need to invest in data cleaning, validation, and infrastructure to ensure the accuracy and reliability of machine learning systems.
Security and Privacy Challenges:
Another important consideration in using machine learning in fintech is the security and privacy of sensitive financial data. Machine learning algorithms require access to large volumes of personal and financial information, which raises concerns about data breaches and unauthorized access. Fintech companies must implement robust security measures and compliance frameworks to protect customer data and ensure regulatory compliance.
How Machine Learning Works
Machine learning is a subset of artificial intelligence that involves using algorithms to enable computer systems to learn from data and make predictions or decisions without being explicitly programmed. In the context of fintech, machine learning algorithms analyze vast amounts of financial data to identify patterns, detect anomalies, and generate insights that can drive smarter financial decisions. Here are the key steps involved in how machine learning works in fintech:
1. Data Gathering: Machine learning algorithms require large datasets to train and learn from. Financial institutions collect data from various sources such as transaction records, customer interactions, market data, and social media.
2. Data Preprocessing: Before using the data for training, it needs to be cleaned, transformed, and standardized to ensure accuracy and consistency. This step involves removing duplicates, handling missing values, and normalizing data.
3. Training the Model: In this step, the machine learning model is trained using the preprocessed data. The algorithm learns from the patterns and relationships in the data to create a mathematical representation that helps make predictions.
4. Testing and Validation: After training, the model is tested using a separate set of data called the validation set. This helps evaluate the model’s performance and ensure that it can generalize well to new, unseen data.
5. Deployment and Predictions: Once the model is trained and validated, it can be deployed in production environments to make predictions or decisions in real-time. These predictions can be used for various financial applications, such as fraud detection, credit scoring, investment recommendations, and personalized customer experiences.
💡 key Takeaway: Machine learning in fintech involves using algorithms to learn from financial data and make predictions or decisions. It follows a process of data gathering, preprocessing, model training, testing, and deployment to enhance various financial services.
Machine Learning and Fintech
Machine learning, a subset of artificial intelligence, has made significant advancements in transforming the financial services industry through fintech. By harnessing the power of algorithms and data, machine learning enables financial institutions to make data-driven decisions, automate processes, and enhance customer experiences.
1. Definition of Machine Learning:
Machine learning refers to the ability of computers to learn and improve from experience without being explicitly programmed. It involves the training of models on large volumes of data, allowing them to identify patterns, make predictions, and continuously optimize their performance.
2. How Machine Learning Works:
In fintech, machine learning algorithms are trained on vast amounts of financial data, including market trends, consumer behavior, and transaction history. These algorithms then analyze and interpret the data, identifying patterns and relationships that might not be obvious to humans. By continuously learning and adapting, machine learning models become more accurate over time.
3. Benefits of Machine Learning in Fintech:
– Enhanced Risk Assessment: Machine learning algorithms can analyze historical data to assess creditworthiness and detect fraudulent activities, allowing financial institutions to make more informed risk decisions.
– Real-time Fraud Detection: By monitoring transactions in real-time, machine learning models can identify unusual patterns or anomalies that may indicate fraudulent activity, helping to prevent potential financial losses.
– Personalized Financial Services: With machine learning, fintech companies can leverage customer data to offer personalized recommendations and tailored financial products, improving customer satisfaction and loyalty.
– Automation and Efficiency: Machine learning enables automation of repetitive tasks, such as data entry and customer support, freeing up human resources to focus on more complex and strategic activities.
4. Examples of Machine Learning in Fintech:
– Robo-advisory services that leverage machine learning algorithms to provide automated investment advice based on individual risk profiles.
– Credit scoring models that utilize machine learning to assess creditworthiness, streamlining loan approval processes.
– Algorithmic trading systems that use machine learning to analyze market data and make automated investment decisions.
5. Lack of Data Quality:
One challenge that arises with machine learning in fintech is the quality and availability of data. High-quality, reliable, and relevant data is crucial for training accurate machine learning models. However, financial data might be fragmented, incomplete, or biased, which can impact the performance and reliability of machine learning algorithms.
6. Security and Privacy Challenges:
As machine learning relies heavily on sensitive financial data, ensuring data security and privacy is critical. Fintech companies need to implement robust security
Benefits of Machine Learning in Fintech
Machine learning has revolutionized the financial services industry by providing numerous benefits and opportunities for innovation. Let’s explore some of the key advantages that machine learning brings to fintech:
1. Accurate Fraud Detection:
Machine learning algorithms can analyze large volumes of financial data in real-time, enabling accurate detection and prevention of fraudulent activities. By continuously learning from patterns and anomalies, machine learning models can flag suspicious transactions, reducing financial losses and enhancing security.
2. Improved Credit Scoring:
Traditional credit scoring models often rely on limited variables to assess a borrower’s creditworthiness. Machine learning, on the other hand, can consider a wide range of data points, including unconventional ones, to build more accurate credit risk models. This allows lenders to make better-informed decisions and offer loans to individuals who may have been overlooked by conventional scoring methods.
3. Personalized Customer Experience:
Machine learning algorithms can analyze customer data, such as transaction history, spending patterns, and online behavior, to create personalized recommendations and offers. By tailoring financial products and services to the individual needs and preferences of customers, fintech companies can enhance customer satisfaction and loyalty.
4. Automated Customer Support:
Thanks to natural language processing and machine learning techniques, chatbots and virtual assistants have become increasingly efficient in handling customer queries and providing support. These AI-powered assistants can quickly respond to customer inquiries, access relevant information, and offer personalized assistance, improving customer service while reducing operational costs.
5. Enhanced Risk Management:
Machine learning enables fintech companies to identify and mitigate potential risks more effectively. By analyzing vast amounts of financial and non-financial data, machine learning algorithms can identify patterns and trends that may signal future market volatility, credit default risks, or economic downturns. This allows financial institutions to proactively manage risks and make more informed investment decisions.
💡 key Takeaway: Machine learning brings several benefits to the fintech industry, including accurate fraud detection, improved credit scoring, personalized customer experiences, automated customer support, and enhanced risk management. These advancements not only streamline financial processes but also lead to better customer satisfaction and trust in financial services.
Examples of Machine Learning in Fintech
1. Fraud Detection and Prevention:
Machine learning algorithms are widely used in the financial industry to detect and prevent fraudulent activities. These algorithms analyze vast amounts of data, including customer transactions, patterns, and behaviors, to identify suspicious activities in real-time. By continuously learning from new data, machine learning models can enhance fraud detection accuracy and reduce false positives, saving financial institutions significant time and resources.
2. Credit Risk Assessment:
Machine learning algorithms help financial institutions in assessing credit risk accurately. By analyzing historical data such as credit scores, payment history, and loan applications, machine learning models can provide more precise credit risk evaluations. This allows lenders to make informed decisions about loan approvals, interest rates, and loan amounts, ultimately leading to more efficient and fair lending practices.
3. Personalized Financial Recommendations:
Machine learning algorithms can analyze vast amounts of customer data to provide personalized financial recommendations. By considering factors such as income, spending habits, financial goals, and risk appetite, these algorithms can suggest tailored investment options, savings plans, and insurance coverage. This level of personalization improves customer engagement and satisfaction, as it demonstrates a deeper understanding of their financial needs.
4. Algorithmic Trading:
Machine learning plays a crucial role in algorithmic trading, where automated systems make investment decisions based on market data and trends. By applying complex mathematical models and real-time data analysis, machine learning algorithms can identify patterns and make high-frequency trades with minimal human intervention. This not only improves trading efficiency but also enables financial institutions to capitalize on market opportunities more effectively.
💡 key Takeaway: Machine learning has revolutionized the financial industry through various applications in fintech. From fraud detection to credit risk assessment and personalized recommendations, these algorithms have enhanced financial services, improved customer experiences, and enabled faster and more efficient decision-making in the industry.
Challenges of Machine Learning in Fintech
Machine learning has undoubtedly revolutionized the financial services industry through fintech, but it also comes with its own set of challenges. Financial institutions face several hurdles when implementing machine learning models in their operations. Here are some of the main challenges:
1. Lack of Data Quality: Machine learning models heavily rely on accurate and high-quality data to make accurate predictions and decisions. However, financial data can be incredibly complex, diverse, and often inconsistent. Poor data quality, such as missing or incorrect information, can significantly impact the performance and reliability of machine learning algorithms.
2. Security and Privacy Concerns: As machine learning systems analyze vast amounts of sensitive financial data, security and privacy become critical concerns. Financial institutions must ensure that the data they use is adequately protected from unauthorized access, breaches, or misuse. Adequate encryption, secure data storage, and compliance with data protection regulations are essential for maintaining the trust of customers and safeguarding their financial information.
3. Regulatory Compliance: Financial services operate within a highly regulated environment to ensure fairness, transparency, and consumer protection. Machine learning models must align with legal requirements, industry standards, and compliance guidelines. Institutions must carefully navigate regulatory frameworks to ensure that their machine learning initiatives meet the necessary legal and ethical standards.
4. Ethical Considerations: Machine learning algorithms need to make decisions that are fair, unbiased, and devoid of any discriminatory tendencies. Bias in machine learning can arise from biased data inputs or biased algorithms. Financial institutions need to be vigilant and continually monitor their models to mitigate any potential bias, ensuring that the decisions made by machine learning systems are fair and equitable for all customers.
5. Interpretability and Explainability: As machine learning models become more complex and sophisticated, it becomes increasingly challenging to interpret how a particular decision was reached. In the financial industry, it is crucial to understand the factors influencing various predictions or decisions made by machine learning algorithms. Explainable AI techniques are being developed to enhance the transparency and interpretability of machine learning models in finance.
💡 key Takeaway: While machine learning brings numerous benefits to the fintech industry, it also poses challenges related to data quality, security, regulatory compliance, ethical considerations, and interpretability. Financial institutions must address these challenges to fully harness the potential of machine learning and ensure its responsible and effective implementation in enhancing financial services.
Lack of Data Quality
One of the primary challenges that machine learning faces in the fintech industry is the lack of data quality. While machine learning algorithms depend on large volumes of high-quality data to make accurate predictions and insights, the financial sector often struggles with incomplete, unreliable, or inconsistent data. Financial systems are complex, with data coming from various sources, and data quality issues can arise due to human error, outdated systems, or lack of data standardization.
To overcome the challenge of data quality, fintech companies need to invest in data cleaning, data validation, and data governance processes. By ensuring the accuracy, completeness, and consistency of data, machine learning algorithms can produce more reliable results. Implementing data quality measures involves data profiling, data cleansing, and validation techniques, such as outlier detection and data integrity checks.
Moreover, collaboration with trusted data providers and leveraging external data sources can enhance the quality and variety of data used for machine learning in the financial sector. Access to accurate and comprehensive data enables machine learning algorithms to make more accurate predictions, identify patterns, and derive meaningful insights to support decision-making processes.
Overall, addressing the issue of data quality is crucial for the successful implementation and utilization of machine learning in fintech. By investing in data cleaning, validation, and governance processes, financial institutions can enhance the reliability and effectiveness of machine learning algorithms, paving the way for more efficient and accurate financial services.
💡 key Takeaway: The lack of data quality is a significant challenge for machine learning in fintech. Investing in data cleaning, validation, and governance processes can enhance the reliability of machine learning algorithms and enable more accurate predictions and insights.
Security and Privacy Challenges
Machine learning has revolutionized the financial industry, particularly in fintech, by enabling businesses to process vast amounts of data and make accurate predictions. However, with this great leap forward comes an array of security and privacy challenges that need to be addressed.
1. Data Security: The increased reliance on data for machine learning algorithms raises concerns about the security of sensitive financial information. Organizations must implement robust security measures to protect data from unauthorized access or breaches.
2. Privacy Concerns: As machine learning algorithms analyze vast quantities of user data, privacy becomes a critical consideration. Fintech companies need to ensure that customer data is handled in a transparent and compliant manner, taking into account regulations like the General Data Protection Regulation (GDPR).
3. Model Bias and Fairness: Machine learning models are only as good as the data they are trained on. Bias in training data can lead to discriminatory outcomes or unfair practices. Fintech companies must actively mitigate bias and ensure fairness in their algorithms to avoid unintended consequences.
4. Adversarial Attacks: Malicious entities can exploit vulnerabilities in machine learning models through adversarial attacks. These attacks deceive the algorithms, leading to incorrect predictions or fraudulent activities. Organizations need to develop robust defense mechanisms to detect and prevent such attacks.
5. Regulatory Compliance: Fintech companies operate within a complex regulatory landscape. Implementing machine learning solutions requires ensuring compliance with financial regulations, data protection laws, and industry-specific requirements. Organizations must navigate these regulations while leveraging the benefits of machine learning.
💡 key Takeaway: Machine learning in fintech brings significant security and privacy challenges that require robust measures to protect sensitive data, address bias, prevent adversarial attacks, and ensure compliance with relevant regulations.
What is the Future of Machine Learning in Fintech
Machine learning is transforming the landscape of the financial services industry, enabling fintech companies to revolutionize how they operate. As the technology continues to evolve, it is expected to play an even more integral role in enhancing financial services. Here are some key aspects that highlight the future potential of machine learning in fintech:
1. Advanced Fraud Detection and Prevention: Machine learning algorithms can analyze vast amounts of data to detect patterns and anomalies, helping financial institutions detect and prevent fraudulent activities in real-time. This not only safeguards the financial system but also protects customers from falling victim to fraud. As financial crimes become more sophisticated, machine learning will continue to strengthen security measures.
2. Personalized Financial Services: Machine learning algorithms can analyze customer data, including spending habits, investment patterns, and risk tolerance, to provide personalized financial advice and tailored product recommendations. This not only improves customer experiences but also enables fintech companies to offer innovative solutions that meet individual needs.
3. Automated Risk Assessment:Machine learning models can automate the risk assessment process, accurately predicting creditworthiness and determining the likelihood of default. This enables financial institutions to make informed lending decisions quickly and efficiently, reducing the risk of default while improving access to credit for individuals and businesses.
4. Enhanced Trading Strategies:Machine learning algorithms can analyze market data, news sentiment, and historical trends to develop powerful trading strategies. These algorithms can identify patterns and correlations that may not be apparent to human traders, ultimately leading to more successful and profitable trades.
5. Efficient Regulatory Compliance: Machine learning can streamline compliance processes by automating tasks such as monitoring transactions for suspicious activities, ensuring adherence to regulatory requirements, and flagging potential violations. This not only reduces the burden on compliance teams but also minimizes the risk of non-compliance.
6. Improved Customer Support: Machine learning-powered chatbots and virtual assistants can provide instant and accurate responses to customer inquiries, offering 24/7 support. These AI-powered assistants can understand natural language, solve problems, and provide guidance, significantly improving customer satisfaction and reducing the need for human intervention.
Machine learning is undoubtedly shaping the future of fintech, bringing efficiency, innovation, and improved customer experiences. As the technology continues to advance, its impact on financial services will become even more pronounced.
💡 key Takeaway: The future of machine learning in fintech holds immense potential for advanced fraud detection, personalized financial services, automated risk assessment, enhanced
Autonomous Financial Services
In the realm of financial technology (fintech), machine learning is revolutionizing the way services are provided, giving rise to the concept of autonomous financial services. By harnessing the power of advanced algorithms and data analysis, machine learning enables financial institutions to automate various processes, making them more efficient and reliable.
1. Enhancing Decision-Making:
Machine learning algorithms have the capability to analyze vast amounts of financial data with lightning speed, helping financial institutions make more accurate and informed decisions. These algorithms can quickly identify patterns, trends, and anomalies in the data, allowing for real-time risk assessment and fraud detection. As a result, autonomous financial services can significantly reduce the likelihood of errors and enhance the overall accuracy of decision-making processes.
2. Personalized Financial Advice:
Machine learning also empowers financial institutions to provide personalized financial advice to their customers. By analyzing individual financial behaviors, spending patterns, and investment preferences, machine learning algorithms can tailor advice and recommendations specifically to each customer’s needs and goals. This level of customization helps customers optimize their financial decisions and achieve their desired outcomes.
3. Efficient Operations:
Autonomous financial services leverage machine learning to automate routine tasks and streamline operational processes. From customer onboarding and loan application processing to regulatory compliance checks, machine learning algorithms can handle these tasks with greater speed and accuracy. This not only saves time and manpower but also enhances operational efficiency, allowing financial institutions to focus on more complex and value-added activities.
4. Fraud Detection and Prevention:
The advanced capabilities of machine learning algorithms make them ideal for tackling the ever-growing threat of financial fraud. By continuously analyzing transactional and behavioral data, these algorithms can detect suspicious patterns and identify potential fraudulent activities. This proactive approach enables early intervention, minimizing the financial losses and reputational damage caused by fraud.
💡 key Takeaway: Autonomous financial services powered by machine learning are transforming the fintech landscape by enhancing decision-making, providing personalized financial advice, streamlining operations, and strengthening fraud detection and prevention.
Improved Customer Experiences
In the realm of financial services, the integration of machine learning has proven to be a game-changer, not only for businesses but also for customers. By leveraging advanced algorithms and data analytics, machine learning has revolutionized the way financial services are delivered, resulting in enhanced customer experiences. Let’s explore some of the key ways in which machine learning contributes to these improvements:
1. Personalized Recommendations: Machine learning algorithms analyze vast amounts of customer data to understand individual preferences, behaviors, and patterns. This enables financial institutions to offer personalized product recommendations and tailored financial advice to customers. For example, based on previous transactions and spending habits, a customer may receive suggestions for budgeting tools or investment options that align with their financial goals.
2. Fraud Detection and Prevention: One of the biggest concerns for customers in the financial sector is the security of their transactions and personal information. Machine learning algorithms are trained to detect anomalous patterns in real-time, enabling rapid identification of potential fraud attempts. By continuously learning from past instances of fraudulent activities, these algorithms can improve their accuracy over time, providing customers with a high level of protection against cyber threats.
3. Seamless Customer Support: Machine learning-powered chatbots have become commonplace in customer support departments. These intelligent bots are capable of understanding and responding to customer queries and concerns in real-time. By utilizing natural language processing and sentiment analysis, these chatbots can provide efficient and accurate solutions, improving the overall customer experience and reducing waiting times.
4. Predictive Analytics: Machine learning algorithms can analyze vast amounts of historical data to predict customer behavior and market trends. This enables financial institutions to design targeted marketing campaigns, offer personalized promotions, and develop innovative products tailored to customer needs. By understanding customer preferences and anticipating their requirements, financial services providers can establish long-term relationships with customers and enhance their loyalty.
5. Enhanced Risk Assessment: With machine learning, financial institutions can better assess creditworthiness and manage risks. By analyzing diverse data points, such as credit history, employment records, and social media activities, machine learning algorithms can generate accurate risk profiles for individuals and businesses. This allows for more informed decision-making in loan approvals and insurance underwriting, resulting in fairer outcomes and reduced default rates.
💡 key Takeaway: Machine learning in fintech has significantly improved customer experiences by enabling personalized recommendations, enhancing security, providing seamless customer support, facilitating predictive analytics, and enhancing risk assessment capabilities. (Explanation): This section is highly relevant to the header “Improved Customer Ex
Conclusion
Machine learning is playing an increasingly important role in the enhancement of financial services through fintech. It helps to improve the customer experience by automating tasks that would traditionally be done by humans. This can result in increased efficiency and decreased costs. Moreover, machine learning can also be used to identify financial trends and patterns. This can help to improve the accuracy of financial predictions and forecasts. In the future, machine learning will likely play an even more important role in the enhancement of financial services. As the technology becomes more advanced, it will be able to perform even more complex tasks.
FAQ
What is the role of machine learning (ML) in the context of fintech?
Machine learning in fintech involves the use of algorithms and statistical models to enable computers to learn from data, make predictions, and improve decision-making processes within the financial services sector.
How does machine learning contribute to fraud detection in financial services?
Machine learning enhances fraud detection by analyzing patterns and anomalies in transaction data. ML algorithms learn from historical data to identify unusual behaviors, helping financial institutions detect and prevent fraudulent activities.
In what ways does machine learning impact credit scoring and lending decisions?
Machine learning is utilized in credit scoring to assess creditworthiness by analyzing diverse data points. It enhances the accuracy of lending decisions by considering a broader range of factors beyond traditional credit history.
Can you explain how machine learning is applied in algorithmic trading and investment strategies?
Machine learning is used in algorithmic trading to analyze market data, identify trends, and execute trades at optimal times. It helps in developing sophisticated investment strategies by learning from historical market behavior.
How does machine learning contribute to personalized financial recommendations and services?
Machine learning analyzes user behavior, preferences, and financial data to provide personalized financial recommendations. This includes customized investment advice, tailored product offerings, and personalized budgeting suggestions.