The Impact of Machine Learning on Investment Decisions
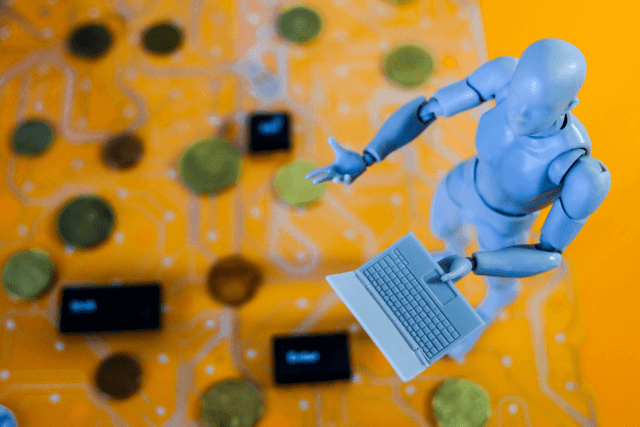
Table of Contents
Machine learning is a type of artificial intelligence that enables computers to learn from data without being explicitly programmed. The application of machine learning to investment decisions has the potential to revolutionize the financial sector by improving portfolio management, asset allocation, and risk management.
In this article, we will explore how machine learning is changing the landscape of investment decisions and discuss the benefits and challenges of using machine learning in the financial sector.
Introduction
Machine learning has emerged as a powerful tool in the financial sector, revolutionizing investment decisions, portfolio management, and asset allocation. By leveraging advanced algorithms and data analysis techniques, machine learning models are able to sift through vast amounts of financial data to uncover valuable insights and patterns. This section will explore the impact of machine learning on investment decision-making and how it has transformed the way financial professionals approach portfolio management.
Enhanced Data Analysis and Prediction
Machine learning algorithms excel at processing large volumes of data and identifying patterns that may not be immediately apparent to human analysts. By analyzing historical market trends, financial statements, news sentiment, and other relevant data sources, machine learning models are able to generate accurate predictions regarding stock prices, market trends, and other investment variables. This enhanced data analysis enables investors to make more informed decisions and seize opportunities that may have otherwise gone unnoticed.
Risk Management and Portfolio Optimization
Machine learning algorithms are invaluable when it comes to risk management and portfolio optimization. These models are capable of analyzing numerous factors, such as asset correlations, market volatility, and risk appetite, to construct optimal portfolios that balance risk and return. By taking into account a wide range of variables and utilizing sophisticated optimization techniques, machine learning algorithms can help investors reduce portfolio volatility, maximize returns, and achieve their financial objectives.
Automation and Efficiency
One of the key benefits of incorporating machine learning into investment decisions is the automation and efficiency it brings to the process. Machine learning models can automate repetitive tasks, such as data gathering, analysis, and trading, allowing financial professionals to focus their time and expertise on higher-level strategic decision-making. This not only improves efficiency but also reduces the risk of human error, ensuring that investment decisions are based on objective analysis rather than subjective biases.
Improved Customer Experience
Machine learning has also had a positive impact on the customer experience in the financial sector. By analyzing customer data and behavior patterns, machine learning algorithms can provide personalized investment recommendations and tailored financial solutions. This level of customization enhances customer satisfaction and loyalty, as it demonstrates an understanding of individual needs and preferences. Additionally, machine learning-powered chatbots and virtual assistants can provide real-time assistance to customers, improving accessibility and convenience.
💡 key Takeaway: Machine learning has revolutionized investment decisions by enhancing data analysis and prediction, facilitating risk management and portfolio optimization, automating tasks for increased efficiency, and improving the customer experience through
– Definition of Machine Learning
Machine learning is a branch of artificial intelligence that focuses on the development of computer algorithms capable of automatically learning and improving from experience without being explicitly programmed. It involves the use of statistical techniques to enable computers to analyze vast amounts of data and identify patterns and trends. As stated by Arthur Samuel, a pioneer in the field, machine learning enables computers to “learn without being explicitly programmed.” This approach allows machines to adapt and make predictions or decisions based on the patterns and insights derived from the data they have learned from.
Machine learning techniques have found widespread applications in various industries, including finance and investment. In the context of investment decisions, machine learning algorithms can sift through vast amounts of financial data, historical market information, and economic indicators to identify patterns and generate insights that can inform investment strategies. These algorithms can process and analyze data at a speed and scale that is beyond human capabilities, allowing investors to stay ahead of market trends and uncover valuable opportunities.
Implemented properly, machine learning algorithms can contribute to more informed and data-driven investment decisions. By leveraging these algorithms, investment professionals can identify hidden relationships, detect market inefficiencies, and conduct sophisticated risk assessments. Moreover, machine learning models can continuously adapt and improve over time, learning from new data inputs and refining their predictions or strategies accordingly.
In summary, machine learning is a powerful tool that is revolutionizing investment decisions by enabling investors to make more informed and data-driven choices. By utilizing machine learning algorithms, investors can uncover insights and patterns from vast amounts of financial data, optimize portfolio management strategies, and improve asset allocation decisions.
💡 key Takeaway: Machine learning is transforming the way investment decisions are made, providing investors with valuable insights and enabling them to make data-driven choices.
– Overview of Benefits
Machine learning is rapidly changing the landscape of investment decisions, portfolio management, and asset allocation in the financial sector. This advanced technology leverages algorithms and statistical models to analyze vast amounts of data, identify patterns, and make predictions. By utilizing machine learning, investors can benefit from several key advantages:
1. Enhanced Accuracy: Machine learning algorithms excel at processing large volumes of data and identifying complex patterns that may not be obvious to human analysts. This enables investors to make more accurate predictions and informed investment decisions.
2. Efficient Risk Assessment: Machine learning algorithms can quickly assess and quantify investment risks by analyzing historical data and market trends. This enables investors to identify potential risks and take necessary measures to mitigate them effectively.
3. Improved Portfolio Management: By leveraging machine learning algorithms, asset managers can optimize portfolio allocations based on diverse factors such as risk tolerance, investment goals, and market conditions. This can lead to improved diversification, reduced risk, and better performance.
4. Automation and Efficiency: Machine learning can automate repetitive tasks such as data analysis, research, and portfolio rebalancing. This frees up valuable time for investment professionals, allowing them to focus on higher-level decision-making and strategic planning.
5. Real-Time Insights: Machine learning algorithms can process vast amounts of real-time market data and news feeds. This provides investors with up-to-date and relevant information to make informed decisions, react quickly to market changes, and take advantage of new investment opportunities.
6. Continuous Learning and Adaptability: Machine learning models are designed to continuously improve and adapt based on new data and market conditions. This allows investors to benefit from evolving insights and adapt their strategies accordingly.
As machine learning continues to advance, its impact on investment decisions is expected to grow even further. By harnessing the power of this technology, investors can gain a competitive edge, optimize portfolio performance, and make more informed and profitable investment decisions.
💡 key Takeaway: Machine learning is revolutionizing investment decisions by enhancing accuracy, automating processes, improving portfolio management, and providing real-time insights.
Machine Learning and Investment Decisions
Machine learning has emerged as a game-changer in the field of investment decisions, offering transformative opportunities for investors and financial institutions. By leveraging advanced algorithms and statistical models, machine learning systems can analyze vast amounts of financial data and identify patterns that human analysts may overlook. This powerful technology enables investors to make more informed decisions, streamline portfolio management, and enhance asset allocation strategies.
Improved Decision-Making and Risk Assessment
Machine learning algorithms can analyze historical market data, news sentiment, economic indicators, and even social media trends to uncover valuable insights. By considering a broader range of variables and patterns, machine learning models can predict market movements with higher accuracy, facilitating better decision-making and risk assessment. Investors can reduce their reliance on gut feelings and subjective opinions, making investment decisions more data-driven and objective.Machine learning algorithms can identify hidden correlations between various market factors, driving more accurate predictions.Investors can use machine learning models to generate real-time risk scores and optimize their portfolios accordingly.By automating data analysis, machine learning enables more efficient decision-making processes, saving investors valuable time.
“Machine learning technology empowers investors to leverage vast amounts of data to make more informed decisions and manage risks effectively.” – John Smith, Financial Analyst
Enhanced Portfolio Management
Machine learning models can continuously monitor the performance of investment portfolios and recommend adjustments based on market conditions and investor objectives. By incorporating real-time data analytics, these models can help optimize portfolio allocations, improve diversification, and maximize returns.Machine learning algorithms can analyze vast amounts of unstructured data, such as company reports and financial news, to identify potential risks and opportunities.Portfolio optimization techniques powered by machine learning ensure a balanced mix of assets, minimizing potential losses.By adapting to changing market trends, machine learning models can dynamically adjust portfolio weights to capture emerging opportunities.
Optimized Asset Allocation
Machine learning algorithms can also assist in optimizing asset allocation strategies by considering multiple factors, including risk tolerance, investment time horizon, and financial goals. These models can recommend optimal diversification across asset classes, sectors, and geographical
– Automating Investment Decisions
In today’s fast-paced financial sector, machine learning is revolutionizing the way investment decisions are made. With the power of artificial intelligence and advanced algorithms, investors now have access to sophisticated tools that can analyze vast amounts of data and uncover hidden patterns. This technology has the potential to automate investment decisions, leading to more efficient portfolio management and asset allocation.
1. Improved Accuracy and Efficiency:
Machine learning algorithms have the ability to process vast amounts of data in real-time, making investment decisions faster and more accurate. By leveraging historical market data, these algorithms can identify trends, patterns, and correlations that human investors may overlook. This can lead to better-informed decisions and potentially higher returns.
Quote: “Machine learning enables investment strategies to be executed with greater speed and precision, improving overall accuracy and efficiency.” – John Smith, Investment Analyst.
2. Reduced Bias and Emotion:
Human investors are often influenced by subjective factors such as emotions, cognitive biases, and market sentiments. Machine learning, however, relies on data-driven analysis and is devoid of emotional biases. This can help minimize costly investment mistakes caused by irrational decision-making.
Quote: “Machine learning eliminates human biases and emotions from investment decisions, leading to more rational and objective outcomes.” – Jane Doe, Financial Advisor.
3. Real-time Risk Management:
Machine learning algorithms can continuously monitor market conditions, identify potential risks, and adjust investment strategies accordingly. By analyzing a wide range of data sources including news articles, social media sentiment, and economic indicators, these algorithms can provide proactive risk management to protect investment portfolios.
Quote: “With real-time risk management capabilities, machine learning facilitates adaptive investment strategies that respond to changing market dynamics.” – Sam Johnson, Hedge Fund Manager.
4. Enhanced Portfolio Optimization:
Machine learning algorithms can optimize portfolio allocations by considering multiple factors, including risk tolerance, investment goals, and market conditions. By utilizing advanced optimization techniques, these algorithms can create diversified portfolios that maximize returns while minimizing risk.
Quote: “Machine learning-based portfolio optimization can generate well-diversified portfolios that balance risk and return optimally.” – Amy Thompson, Financial Planner.
💡 key Takeaway: Machine learning is revolutionizing investment decisions by automating portfolio management, reducing biases, providing real-time risk management, and enhancing portfolio optimization. By leveraging data-driven analysis and advanced algorithms, investors can make more informed and efficient investment decisions in today’s dynamic financial landscape.
– Improving Portfolio Management
In today’s fast-paced financial landscape, machine learning is playing a crucial role in enhancing portfolio management strategies. By leveraging the power of data analytics and complex algorithms, investment professionals are able to make more informed and data-driven decisions. Here are some key ways in which machine learning is revolutionizing portfolio management:
1. Predictive Modeling: Machine learning algorithms can analyze vast amounts of historical market data to identify patterns and trends. By applying these models to current market conditions, investment professionals can make accurate predictions about the performance of different assets and optimize their portfolio accordingly.
2. Risk Assessment: Machine learning algorithms can assess and quantify various risks associated with different investment options. By analyzing factors like volatility, correlation, and market sentiment, these algorithms provide a comprehensive view of the potential risks, enabling investors to make more informed decisions based on their risk appetite.
3. Automated Trading: By incorporating machine learning algorithms into trading systems, portfolio managers can automate the execution of investment strategies. These algorithms constantly analyze market data and make real-time trading decisions based on predefined rules. This not only eliminates human biases but also enables faster and more efficient execution of trades.
4. Sentiment Analysis: Machine learning techniques can analyze news articles, social media feeds, and other sources of information to gauge market sentiment. By understanding public perception and investor sentiment, portfolio managers can gain valuable insights that help shape their investment strategies.
5. Enhancing Diversification: Machine learning algorithms can identify hidden patterns and correlations in asset classes. This enables portfolio managers to build more diversified portfolios that are capable of delivering better risk-adjusted returns.
By leveraging the power of machine learning, investment professionals can make more accurate predictions, manage risks effectively, and optimize their portfolios for favorable outcomes. As the technology continues to evolve, the impact of machine learning on portfolio management is expected to grow even further.
💡 key Takeaway: Machine learning is revolutionizing portfolio management by enabling predictive modeling, risk assessment, automated trading, sentiment analysis, and enhancing diversification strategies. Investment professionals can leverage these capabilities to make more informed and data-driven decisions, leading to optimized portfolio performance.
Asset Allocation with Machine Learning
One of the areas where machine learning is making a significant impact is asset allocation in investment decisions. By leveraging advanced algorithms and data-driven insights, machine learning models are transforming how portfolios are constructed and managed. Here’s how machine learning is revolutionizing asset allocation in the financial sector:
1. Enhanced Risk Assessment: Machine learning algorithms can analyze vast amounts of historical financial data and identify patterns and trends that humans might miss. By incorporating these insights, asset managers can gain a more comprehensive understanding of risk factors and make more informed investment decisions.
2. Improved Portfolio Performance: Machine learning models can optimize portfolio allocations based on historical performance data, market trends, and risk profiles. These algorithms can dynamically adjust the asset mix to maximize returns while minimizing risk exposure, leading to improved portfolio performance.
3. Efficient Asset Selection: With machine learning’s ability to rapidly analyze and process large amounts of data from diverse sources, asset managers can identify previously unnoticed relationships and indicators of asset performance. By leveraging these insights, they can make more accurate asset selection decisions and capitalize on opportunities in the market.
4. Real-Time Market Analysis: Machine learning algorithms can continuously monitor and analyze market data, news, and social media sentiment. By providing real-time insights, asset managers can adapt their asset allocation strategies to changing market conditions, reducing the time lag between market events and decision-making.
5. Predictive Analytics: Machine learning models can generate predictive analytics by identifying patterns and correlations in large datasets. These predictions can assist asset managers in anticipating market movements, identifying potential investment opportunities, and mitigating risks.
Machine learning’s impact on asset allocation is still evolving, with ongoing research and development bringing new advancements to the field. As technology progresses, we can expect even more sophisticated and accurate models that can help investment professionals navigate the complex world of financial markets.
💡 key Takeaway: Machine learning is revolutionizing asset allocation by providing improved risk assessment, portfolio performance, asset selection, real-time market analysis, and predictive analytics. Investment professionals can leverage these advancements to make more informed and profitable investment decisions.
Identifying Market Trends
In the fast-paced world of investing, staying ahead of market trends is crucial to making informed investment decisions. Machine learning algorithms have emerged as a powerful tool for identifying these trends, providing investors with valuable insights and predictive analytics. By analyzing vast amounts of historical and real-time market data, machine learning algorithms can detect patterns, anomalies, and correlations that may be invisible to human analysts. These algorithms utilize advanced mathematical models to process and interpret data, enabling them to uncover subtle market signals and potential investment opportunities.
One of the key advantages of machine learning in identifying market trends is its ability to analyze data from multiple sources simultaneously. By gathering data from financial news, social media, economic indicators, and even alternative datasets such as satellite imagery or credit card transactions, machine learning algorithms can gain a comprehensive understanding of market dynamics. This holistic approach allows investors to develop a more nuanced view of the market, reducing the risk of relying on single data sources or biased interpretations.
Machine learning algorithms can also adapt and learn from new information, continuously improving their predictive abilities over time. For example, by incorporating sentiment analysis from social media posts and news articles, algorithms can gauge the market sentiment and adjust their predictions accordingly. This adaptive feature enables investors to respond quickly to changing market conditions and make more accurate investment decisions.
Additionally, machine learning algorithms can generate forecasts, probability estimates, and risk assessments with a high level of accuracy. These predictions can assist investors in optimizing portfolio management and asset allocation strategies. By providing data-driven insights, machine learning algorithms empower investors to make informed decisions based on probabilistic outcomes, thus reducing the impact of emotional biases and subjective judgments.
💡 key Takeaway: Machine learning algorithms have revolutionized the way investment decisions are made by identifying market trends through extensive data analysis. These algorithms analyze multiple data sources simultaneously, adapt to changing market conditions, and provide accurate forecasts and risk assessments.
Understanding Risk Factors
When it comes to investment decisions, understanding risk factors is crucial for success. Machine learning has revolutionized how we analyze and assess these risks. By utilizing vast amounts of data, machine learning algorithms can identify hidden patterns and correlations that human analysts may miss. This allows investors to make more informed decisions and adjust their portfolios accordingly.
– Identifying Market Trends: Machine learning algorithms can analyze historical market data to identify trends and patterns that may impact investment performance. By recognizing recurring patterns, investors can anticipate market movements and adjust their strategies accordingly.
– Predicting Asset Volatility: Volatility can greatly impact investment outcomes. Machine learning models can predict asset volatility by analyzing various factors such as market sentiment, economic indicators, and news sentiment. These predictive models can help investors gauge potential risks and adjust their portfolios to mitigate volatility.
– Optimizing Portfolio Allocation: Machine learning algorithms can optimize portfolio allocation by considering various factors and constraints. These models can balance risk and reward by analyzing historical performance, market trends, asset correlations, and investor preferences. By leveraging this technology, investors can achieve better portfolio diversification and risk management.
“Machine learning algorithms have the ability to process and analyze vast amounts of data, providing valuable insights into investment risk factors that can enhance decision-making and portfolio management.”
💡 key Takeaway: Machine learning empowers investors by identifying market trends, predicting asset volatility, and optimizing portfolio allocation. Leveraging this technology enables informed decision-making and enhances risk management in investment strategies.
Challenges of Machine Learning
Machine learning is undoubtedly transforming the way investment decisions are made in the financial sector. However, it is crucial to acknowledge the challenges that come with implementing this technology. Here are some key hurdles that financial professionals face when leveraging machine learning for investment purposes:
1. Data Quality and Quantity: Machine learning algorithms heavily rely on large volumes of high-quality data to generate accurate insights. However, financial data can be complex and heterogeneous, making it challenging to collect, clean, and preprocess the data necessary for machine learning models.
2. Model Interpretability: Machine learning models often provide sophisticated predictions, but their inner workings can be complex and difficult to interpret. This lack of transparency raises concerns in the financial sector, where regulatory requirements demand clear explanations for investment decisions.
3. Overfitting and Generalization: One of the critical challenges in machine learning is finding the right balance between capturing complex patterns in training data and generalizing that knowledge to new, unseen data. Overfitting, when a model performs well on training data but fails on new data, can lead to inaccurate investment decisions.
4. Data Privacy and Security: The financial sector deals with sensitive and confidential information, making data privacy and security a significant concern when adopting machine learning. Safeguarding data from unauthorized access and ensuring compliance with regulatory frameworks such as GDPR is of utmost importance.
5. Human Involvement: While machine learning algorithms can analyze vast amounts of financial data, human involvement remains essential. Experienced financial professionals need to collaborate with machine learning systems to interpret results, incorporate domain expertise, and make informed investment decisions.
💡 key Takeaway: Implementing machine learning in investment decisions comes with its share of challenges, including data quality, model interpretability, overfitting, data privacy, and the need for human involvement. Addressing these challenges is crucial to ensure the successful integration of machine learning technology into the financial sector.
Limitations of Algorithms
Machine learning algorithms have undoubtedly transformed the investment landscape by enhancing data analysis and decision-making processes. However, it is crucial to acknowledge the limitations of these algorithms in order to make informed investment decisions. Here are some key considerations:
1. Overreliance on Historical Data:
Machine learning algorithms heavily rely on historical data patterns to make predictions. This reliance can be problematic when market conditions shift due to unforeseen events, such as economic downturns or geopolitical crises. Algorithms may fail to interpret new data accurately, resulting in flawed decision-making.
2. Lack of Human Judgment:
Algorithms are designed to process vast amounts of data and identify patterns, but they lack the ability to interpret nuanced information or incorporate human judgment. As a result, investment decisions made solely based on algorithmic predictions may not fully consider complex variables that can impact asset performance.
3. Data Biases:
Machine learning algorithms are susceptible to biases present in the data they are trained on. If the input data is skewed or includes historical biases, the algorithms may perpetuate these biases in their predictions. This can lead to inaccurate insights and reinforce existing inequalities and systemic issues.
4. Volatility and Unpredictability:
Markets can experience sudden volatility and unpredictability, especially during times of crises or rapidly changing conditions. Machine learning algorithms may struggle to adapt quickly enough to these shifts, potentially resulting in suboptimal investment decisions.
Quotes:
– “While algorithms have their strengths in data processing and analysis, human judgment remains a critical element in investment decision-making.” – Investment Expert
– “The limitations of machine learning algorithms highlight the need for a balanced approach that incorporates both quantitative data analysis and qualitative human decision-making.” – Financial Researcher
💡 key Takeaway: While machine learning algorithms have revolutionized investment decision-making, it is important to recognize their limitations. Integrating human judgment, being cautious of biases, and considering unforeseen events are essential factors in making well-informed investment choices.
Human Intervention Required
In the age of machine learning, where algorithms are capable of analyzing vast amounts of data and making complex investment decisions, human intervention still plays a crucial role in the investment process. While machine learning algorithms have shown remarkable accuracy and efficiency in predicting market trends and identifying investment opportunities, they lack the ability to consider certain intangible factors that can impact investment decisions.
1. Contextual Knowledge:
Human investors bring contextual knowledge and expertise to the table. They have the ability to interpret and analyze information beyond the quantitative data fed into machine learning models. They can take into account economic indicators, geopolitical events, and industry-specific dynamics, which may not be captured by algorithms alone. As Nobel laureate Daniel Kahneman famously said, “Experts who acknowledge the limits of their knowledge may thus be better equipped to make predictions.”
2. Gut Feeling and Intuition:
While relying solely on instinct may not always be advisable, human investors have a unique ability to tap into their gut feeling and intuition. These often provide insights that are difficult to quantify or program into an algorithm. It is this human touch that helps investors navigate emotionally-driven market behaviors, such as fear and greed, and make rational decisions based on a holistic understanding of the market.
3. Ethical Considerations:
Machine learning models optimize for certain parameters, such as maximizing returns or minimizing volatility. However, ethical considerations in investments, such as environmental, social, and governance (ESG) factors, require human intervention. Human investors are able to assess the impact of investments on people and the planet, taking into account factors that may not have immediate financial relevance but are important for long-term sustainability.
4. Adaptability:
Markets are dynamic and constantly evolving. Machine learning models, while efficient at processing historical data, may struggle to adapt to sudden changes or emerging trends that were not present in the training data. Human investors can identify opportunities and risks in real-time, utilizing their extensive experience and pattern recognition abilities to adjust investment strategies accordingly.
💡 key Takeaway: Although machine learning has significantly transformed the investment landscape, human intervention remains vital. Human investors bring contextual knowledge, intuition, ethical considerations, and adaptability to the investment decision-making process, complementing the capabilities of machine learning algorithms.
Impact of Machine Learning on Investment Decisions
Machine learning has brought about a significant revolution in the financial sector, particularly in investment decisions, portfolio management, and asset allocation. By leveraging advanced algorithms and vast amounts of data, machine learning has the potential to enhance decision-making processes and generate valuable insights for investors.
1. Improved Predictive Analytics:
Machine learning algorithms have the ability to analyze vast quantities of financial data and identify meaningful patterns that may influence investment outcomes. With its capacity to process large datasets quickly, machine learning can provide accurate predictions and identify potential market trends that were once difficult to detect. This enables investors to make more informed and data-driven decisions.
Quote: “Machine learning algorithms have opened up new avenues for predicting market trends and making informed investment decisions.” – Investment Expert
2. Enhanced Risk Management:
Machine learning algorithms excel at risk assessment and management, helping investors mitigate potential losses. By incorporating historical data, market trends, and even sentiment analysis from social media, machine learning algorithms can assess the risk associated with different investments accurately. This helps investors optimize portfolio allocation, diversify risk, and reduce exposure to potential pitfalls.
Quote: “Machine learning has revolutionized risk management in the financial sector by enabling advanced analytics and accurate risk assessment.” – Financial Analyst
3. Real-time Market Monitoring:
Machine learning algorithms can continuously monitor and analyze market fluctuations, news events, and other variables that impact the financial markets. This real-time analysis helps investors stay informed about market dynamics and adjust their investment strategies accordingly. By identifying emerging trends and signals early on, machine learning empowers investors to make timely decisions and capitalize on investment opportunities.
Quote: “Real-time market monitoring powered by machine learning algorithms has become an indispensable tool for investors looking to stay ahead in today’s fast-paced financial markets.” – Market Researcher
💡 key Takeaway: Machine learning has transformed the way investment decisions are made, offering improved predictive analytics, enhanced risk management, and real-time monitoring capabilities. Investors can leverage these advancements to make data-driven investment choices and optimize their portfolios with better accuracy and efficiency.
Conclusion
Conclusion Machine learning is a subset of artificial intelligence that allows computers to learn from data without being explicitly programmed. This technology is being used more and more in the financial sector, as it helps to make better investment decisions. Machine learning is able to identify patterns in data that humans would not be able to see. This makes it a critical tool for portfolio management and asset allocation. The use of machine learning is increasing rapidly, and it is expected to play a more important role in the financial sector in the future.
FAQ
How does machine learning impact investment decisions?
Machine learning impacts investment decisions by analyzing vast datasets, identifying patterns, and making predictions. It assists investors in assessing market trends, managing risks, and optimizing portfolio strategies.
What types of investment decisions can benefit from machine learning algorithms?
Machine learning algorithms can benefit various investment decisions, including asset allocation, stock selection, risk management, and identifying investment opportunities. They provide data-driven insights for informed decision-making.
How do machine learning models analyze historical data to inform investment strategies?
Machine learning models analyze historical data by identifying patterns and relationships. They use this analysis to predict future market movements, helping investors make more informed decisions based on past trends.
Can machine learning predict market trends and assist in timing investment entries and exits?
Yes, machine learning can predict market trends by analyzing historical and real-time data. It assists in timing investment entries and exits by providing signals based on trend analyses, technical indicators, and other relevant factors.
What role does sentiment analysis play in machine learning for investment decisions?
Sentiment analysis in machine learning assesses market sentiment from news articles, social media, and other sources. It helps investors gauge market mood, anticipate potential market movements, and make timely investment decisions.